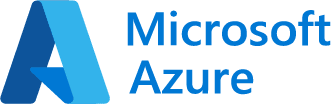
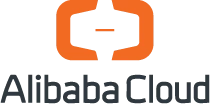
Streamline cloud operations: Migrate, Protect, and Optimize your cloud environment
Thank you for your request!
We will be in touch soon.
We respect your privacy. See our Privacy Policy. You can unsubscribe at any time.
Trusted by
Top use cases
Migrate all types of workloads from any-to-any cloud platform, including VMware to KVM/OpenStack scenario quickly, effortlessly, and securely.
Hystax Acura provides users with a fully automated migration process with consistent background replication and instant launch of workloads on a target site. Network traffic compression and deduplication during replication significantly reduce both the time required for data transfer and the use of cloud resources.
Perform disaster recovery or backup for OpenStack to restore all types of workloads and applications without any data loss and downtime.
With Hystax Acura, companies get full disaster recovery automation. The solution offers users an agentless replication process, low RPO and RTO values, and protected data retention in block devices.
Hystax Acura provides the ability to replicate OpenStack infrastructure settings and recreate them on the OpenStack receiver: tenants, subnets, and flavors.
OptScale, an open source platform, delivers FinOps and cloud cost optimization solutions for all types of organizations. Get hundreds of utilization recommendations, including efficient utilization of RI/SP, unused resource detection, S3 duplicate object finder, resource bottleneck identification, optimal instance type and family selection, R&D resource power management, and rightsizing.
Optimize cloud costs and gain complete visualization of your spending on resource usage in AWS, MS Azure, GCP, Alibaba Cloud, or any Kubernetes cluster.
Thank you for your request!
We will be in touch soon.
We respect your privacy. See our Privacy Policy. You can unsubscribe at any time.
We run a FinOps community with 10,000+ members
Supported platforms
Explore fully automated Hystax Acura software that allows you to securely attain robust IT Resilience and ensure uninterrupted Business Continuity with low RPO and RTO values
Find out how to: