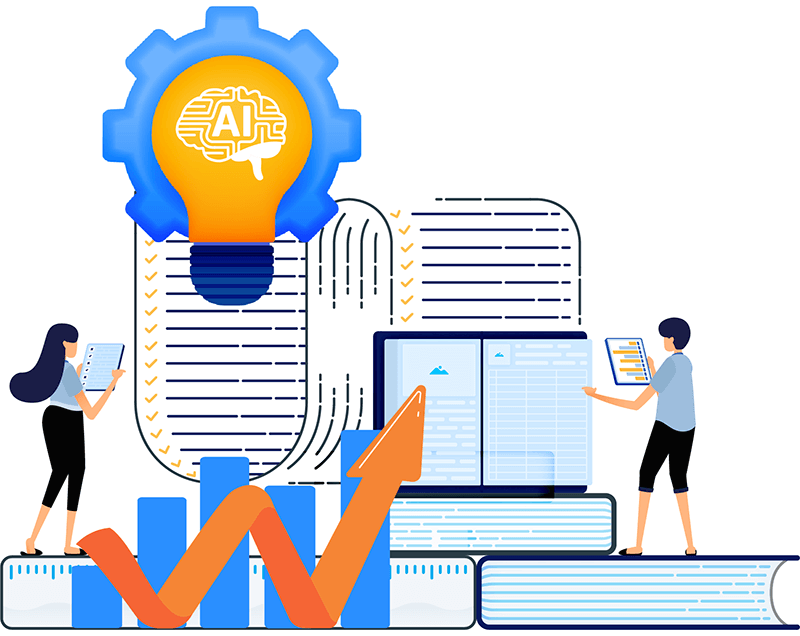
Cloud computing has gained considerable prominence in business due to its capabilities. Still, the effective management of cloud expenses remains a complex endeavor. Nevertheless, incorporating automation and Machine Learning (ML) provides an avenue to control and mitigate cloud costs efficiently, rendering cloud computing a more economically viable solution. This article will delve into the transformative influence of automation and Machine Learning on cloud cost optimization, offering insights into how enterprises can harness these technologies to curtail expenses while addressing potential implementation challenges.
The significance of automation and Machine Learning in managing cloud expenses
The management of cloud costs remains a persistent challenge for companies despite the significant cost-saving benefits of cloud computing. Two pivotal elements in improving cost control are automation and Machine Learning (ML).
Automation for proactive cost management
Automation is crucial in optimizing cloud expenses by constantly monitoring resource usage and responding to deviations automatically. For instance, it can issue alerts or take corrective actions when usage surpasses predefined thresholds. Additionally, automation can help identify and shut down unused resources and move cases to more budget-friendly regions. Moreover, it facilitates the selection of the most cost-effective cloud provider or instance type, ensuring that the company maximizes its cost-efficiency.
ML for data-driven cost precision
Machine Learning algorithms excel in enhancing cost control accuracy by automatically analyzing usage data and uncovering intricate usage trends that might be challenging to identify manually. ML is proficient at identifying anomalies in usage patterns, offering insights into potential cost-saving opportunities. For instance, ML can detect sudden usage spikes, recommend more efficient instance types, or suggest migrating to cost-effective regions or providers. This empowers companies with data-driven insights to make informed decisions and effectively manage their cloud costs.
How can automation contribute to the optimization of cloud costs?
Automation is a crucial asset in pursuing cost optimization within the cloud environment. It significantly reduces the need for manual intervention, mitigates the risk of human errors, and bolsters transparency regarding cloud expenditure. Notable ways in which automation can facilitate the management of cloud expenses encompass:
Precise cost attribution via resource tagging
Automation simplifies the tagging of cloud resources with pertinent cost-related information. This tagging mechanism ensures that cloud costs are accurately tracked and attributed, affording organizations improved transparency and insights into their cloud expenditure.
Dynamic resource scaling
Automation dynamically adjusts cloud resources in response to fluctuations in demand, ensuring that resources are appropriately sized. This responsive approach enhances resource utilization efficiency and leads to tangible cost savings.
Resource monitoring and efficiency enhancement
Automation systems vigilantly oversee cloud resources, promptly identifying instances of inefficient utilization. This process aids in recognizing and eliminating unused or underutilized cloud resources, resulting in substantial cost savings.
The advantages of implementing automation for cloud cost management
Improved visibility
Automation systems play a pivotal role in accurately tracking and attributing cloud costs. This, in turn, results in heightened visibility into the intricacies of cloud-related expenditure, allowing for more informed decision-making and financial management.
Cost reduction
The core objective of automation is to detect and rectify instances of underutilized or redundant cloud resources. Through this proactive approach, automation effectively drives down costs, facilitating substantial savings for organizations.
Integrating automation
Integrating automation into cloud cost control processes offers a significant boost in efficiency. It accomplishes this by diminishing the need for manual intervention, reducing the potential for human errors, and elevating overall accuracy in managing cloud costs.
Understanding Machine Learning
Machine Learning is a facet of artificial intelligence that employs algorithms and data to glean insights from historical experiences, subsequently enabling the generation of predictions or recommendations. It emerges as a potent instrument for cost control by pinpointing and mitigating waste and inefficiencies and identifying avenues for cost savings. Examples of how Machine Learning can effectively govern cloud expenses encompass:
Predictive cost analysis
Machine Learning leverages usage and demand patterns to conduct predictive analyses of cloud expenses, facilitating astute budgeting and resource allocation decisions.
Resource optimization
Machine Learning excels in identifying underutilized resources, providing recommendations to enhance resource utilization, and proposing cost-effective instance types, optimizing cloud resource allocation.
Automated spending oversight
Machine Learning proficiently identifies instances in which spending surpasses anticipated levels and suggests strategies for cost reduction, thereby ensuring that cloud expenditure remains in check.
The merits of employing Machine Learning for cloud cost control
Elevated visibility
Machine Learning augments the visibility into cloud costs, making identifying and resolving cost-related issues more accessible and understandable.
Enhanced efficiency
Machine Learning can automate and streamline cost control procedures, rendering them more facile and efficient than manual methods.
Cost optimization
By discerning and rectifying wasteful practices and inefficiencies, Machine Learning is a catalyst for reducing cloud-related expenses, contributing to considerable cost savings.
Informed decision-making
Machine Learning enriches the analytical process by offering superior insights and more precise cost predictions, enabling organizations to make well-informed decisions that align with their financial objectives.
Navigating the obstacles of employing automation and Machine Learning in cloud cost optimization
Organizations embarking on the journey of cloud cost control via automation and Machine Learning may encounter several challenges during implementation. These challenges include:
- The issue of over or under-provisioning cloud resources is a significant concern, as it can lead to inefficiencies and increased expenses. While automation and Machine Learning provide valuable tools to mitigate this risk, it is essential to have a comprehensive understanding of the cloud environment and resource usage.
- Automating the control of cloud costs requires a deep knowledge of the specific cloud environment and the ability to analyze historical usage data while identifying patterns in current usage. Each instance of automated cost control should be carefully tailored to the unique characteristics of the cloud architecture and the specific usage patterns, as different situations may require distinct approaches.
- Although powerful, Machine Learning algorithms introduce complexities in setup and ongoing maintenance. They require extensive datasets to deliver effective results, requiring meticulous data labeling and organization. This time-consuming process is prone to errors. Furthermore, machine learning algorithms can be financially demanding and require continuous maintenance due to their intricate nature.
Notably, while these challenges may seem daunting, they can be surmounted with the assistance of the right partner. Aspire Systems, for instance, offers customized cloud optimization strategies, which reduce costs, enhance performance, and fortify security. Their experts are well-versed in all major cloud platforms, such as AWS, MS Azure, and GCP, harnessing on-demand and cloud-native services to optimize operational costs. By providing businesses with in-depth insights into cloud resource consumption across departments and teams, enterprises can gain enhanced control over their cloud instances, storage, cost mapping by business unit, and more.
In conclusion
Adopting automation and Machine Learning technologies is reshaping how businesses handle their cloud cost management. These tools offer enhanced visibility into the cloud environment and promise enduring advantages. By harnessing the power of these technologies, companies can make more informed choices. As automation and Machine Learning progress, cloud cost control will evolve, becoming more efficient and potent, enabling businesses to optimize the returns on their cloud investments.
✔️ Do you want your cloud and ML/AI operations to be under control and your expenses to meet your expectations? Assess the capabilities and potential of an open source platform OptScale → https://hystax.com/introducing-optscale-public-release-an-open-source-powerhouse-for-finops-and-mlops/
OptScale Group Demo ‘How to build a FinOps & MLOps process to optimize cloud workload performance and cost’ 📅 September 27th 🕑10 am PST
Join us to discover the difference between FinOps and Cloud Cost Management, which saving recommendations have the biggest impact, how to avoid bill shocks, and how MLOps helps increase the efficiency of ML experiments.
Interested? Sign up here➡️
https://hystax.com/optscale-demo-how-to-build-finops-and-mlops-process-to-optimize-cloud-workload-performance-and-cost