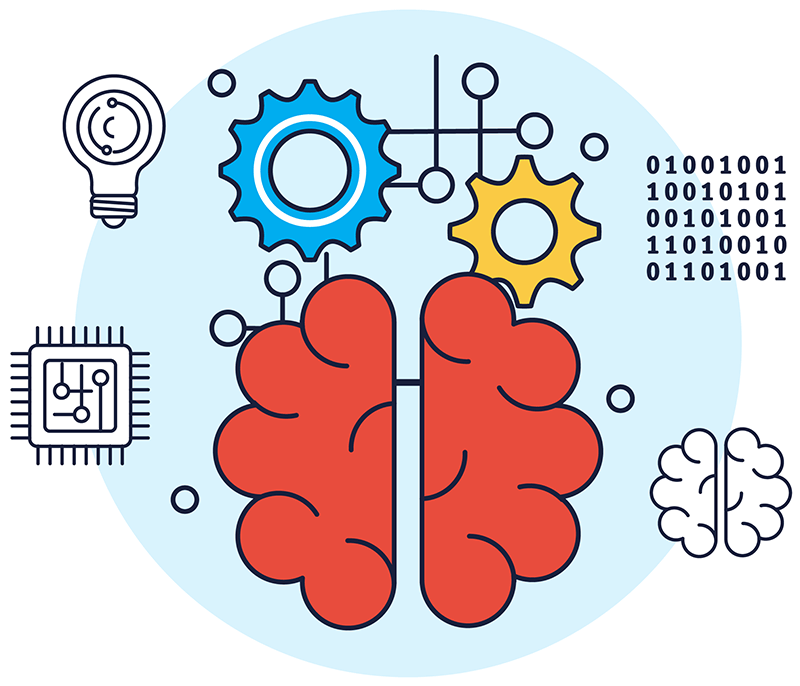
In the ever-evolving landscape of technology, the past decade has witnessed a remarkable shift. With the advent of cloud computing, organizations have been searching for solutions that break free from hardware limitations and embrace dynamic flexibility. Enter the stage: software-defined infrastructure (SDI). This ingenious concept stitches together computing, networking, and storage into a unified tapestry, providing a scalable ecosystem that dances to the tune of growth.
But as the digital tide surges, a fresh challenge emerges on the horizon. The sheer deluge of data has spotlighted the fragility of IT systems – their adaptability, security, and elasticity. The playbook for dealing with the unexpected has morphed, requiring strategies that navigate diverse data streams:
- from the whispers of apps to the clamor of sensors
- from the visualization of dashboards to the intricate pathways of edge networks
The rigid, preset codes of yesteryears show their limitations in this dynamic theatre.
Stepping into this arena with capes billowing are AI-defined infrastructures (ADIs). These aren’t just your ordinary SDIs; they’ve been infused with the magic of self-learning and self-correcting capabilities. Imagine systems that:
- allocate resources based on demand
- arrange components by drawing from past experiences
- anticipate data-based theatrics even before the curtains rise on errors
These superheroes are needed to tame the unruly data jungles where human comprehension falters in the face of overwhelming information.
Think of these algorithms as the stage directors, skillfully curating the performance, focusing attention, and orchestrating most metrics to flow seamlessly without human intervention.
What sets the AI-defined marvels apart is their penchant for self-improvement. Unlike their rule-bound counterparts, these machine-learning maestros can decipher patterns within the data and choreograph responses that waltz with the rhythm of evolving environments. It’s a symphony that offers an elegant alternative to the traditional cacophony of “thresholding” approaches, which often miss nuances or sound false alarms.
The grand entrance of AI-infused architectures couldn’t be timelier for businesses standing at the crossroads. As the costs of cloud computing ascend and privacy concerns heighten, IT leaders are questing for tools to conduct a symphony of resource optimization while keeping costs at a minimum. Enter the virtuoso performance of AI-driven IT infrastructures.
Now, let’s pull back the curtains and spotlight distinct scenarios where their artistry truly shines:
Smart storage handling in the age of data overflow
From data deluge to insightful AI: We live in an age where data pours in non-stop. But it wasn’t always like this. Back then, storage systems were like static libraries, hard-programmed and inflexible. Unfortunately, they struggled to keep up with the dynamic flow of today’s data. This resulted in precious information being lost or left unutilized.
Enter the AI savior: Cue the hero music – AI steps onto the scene to bring order to this chaos. It’s like having a master organizer for your digital mess. AI empowers IT teams to stay on top of constantly changing storage needs. It’s like having a virtual storage manager that knows exactly when to expand, shrink, or reorganize your storage.
Tailoring storage to data lives: But here’s the magic touch – AI creates storage systems that adapt to how data lives and breathes. Imagine your storage like a multi-layer cake, with each layer serving a different purpose. AI makes this cake smart, adjusting the layers based on the data’s “lifecycle” – from freshly baked to a bit stale. It even keeps an eye on the speed at which data goes in and out, ensuring everything runs smoothly.
Efficiency and savings: Let’s talk benefits. This AI-powered storage maestro keeps your data organized and saves you money. It’s like having a thrifty accountant who knows when to splurge and when to save. Using AI’s predictive modeling, the system knows exactly when to adjust storage levels based on how you use it. Old, less-needed data is gently moved to more budget-friendly storage spaces, freeing up prime storage real estate.
Future’s storage symphony: The story doesn’t end here. AI’s role in storage management is just beginning. It’s like the book’s first chapter, where AI learns and grows over time. Imagine even more efficient and effective storage solutions, like leveling up from a beginner to a grandmaster in a game.
Unveiling AI’s storage magic: So, there you have it – the tale of how AI turns storage chaos into a symphony of efficiency and cost savings. It’s like having a tech-savvy conductor orchestrating the perfect storage harmony.
Unveiling unforeseen resource surges
Spotting the unusual: Consider it a detective story in the tech world. Just as we’re smartly managing resources, we’re also training our tech sleuths to spot the odd ones. Anomaly detection is a skill to catch unexpected happenings, whether done by machine learning or coded instructions. The aim? To swiftly recognize crucial events, leading to quicker fixes and shorter downtimes.
AI’s insightful help: Imagine if our machines could spot oddities and explain why they happened. With the help of explainable AI, our Machine Learning models can do just that. It’s like having a detective who solves the mystery and shows you the clues and steps that led to the solution. This real-time insight into the root cause of anomalies prevents service hiccups and false alarms.
Precision through personalization: Now, let’s add a dash of personalization. These AI models are like chameleons – they adapt to your unique situation. By being trained on your organization’s specific data, they become super skilled at detecting issues that matter to you. It’s like having a detective who knows your neighborhood so well that they can spot when something’s off even before you realize it.
The tale of anomaly triumph: So, in the grand narrative of tech prowess, here’s the story of anomaly detection. It’s about having the digital equivalent of Sherlock Holmes on your side – spotting the strange and unexpected and showing you the why and how all in real-time.
Enhancing scalability with AI in IT systems
Adapting to organizational needs: IT departments are tasked with ensuring that networks, databases, and applications can scale according to the organization’s requirements, whatever they may be.
Time-sensitive scalability: The ability of infrastructural capacities to scale in response to changing demands over time is critical to true adaptability.
AI-driven elastic scaling: Instead of relying on manually coded rules, AI-assisted infrastructures can automatically adjust their scale based on demand predictions.
Historical data for informed scaling: Machine learning models analyze historical data on resource demand across different applications, allowing systems to make informed decisions as they scale up or down.
The human element: Scalability isn’t solely a technical consideration. The human factor is vital. “Experiential AI,” or AI with human input, is crucial to incorporate human insights into scalability decisions.
Optimal solution: Integrating AI with human oversight provides the best approach for IT departments to account for the human variable, ensuring scalability aligns with technical and human considerations.
Smarter cloud resource management
Cloud’s resource oasis: Imagine the cloud as a treasure chest of computing power businesses with limited resources can tap into. However, the catch is that the offerings from cloud providers often provide only specific solutions. They create a mixed infrastructure with different speeds, locations, and unpredictable service requests.
Patching the patchwork: Merging these solutions is like piecing together a puzzle with variable pieces. This creates an infrastructure quilt with patches of varying bandwidths, different geographic areas, and random service needs. While it might seem wise to keep adding more cloud services, this strategy can be costly and inefficient.
AI’s crystal ball: But hold on, there’s a more innovative way. Imagine if machines could predict the future – not with a magic crystal ball but with Machine Learning models. These models can foresee resource needs and allocate them in real-time. They’re like weather forecasters, predicting trends and even dealing with sudden storms. So, workloads are juggled based on what resources are available in the system at any given moment.
The human-AI duo: Now, some tech-savvy folks might want to go full throttle and automate everything about resource management. But here’s where the AI experts step in with advice: balance is essential. They suggest a mix of automation and human wisdom. Think of it like having AI-driven helpers who handle everyday tasks, but they also respect your decision-making skills for the tricky stuff. This “human in the loop” approach gives you the best of both worlds.
Saving secrets: The AI magic doesn’t stop there. These algorithms are smart cookies. They learn when to hit the pause button on some parts of the infrastructure and restart later. It’s like knowing when to turn off the lights to save electricity. This trick can lead to considerable savings in computing power.
Two tales of intelligence: So, there you have it, the story of smarter cloud management and scaling. But wait, there’s more! Let’s jump into another tale – one of intelligent scalability.
Embrace the human element: AI's power and human oversight
In the realm of modern technology, the potential of AI-driven IT infrastructure is undeniable. It improves diverse areas such as data collection, content management, network security, server optimization, resource planning, and customer relationship management. The integration of AI, however, comes with a word of caution. While AI systems excel in many tasks, they can exhibit biases and struggle to interpret unusual data accurately when deployed without human guidance.
To strike the right balance, algorithms must actively seek affirmation and feedback from human operators. This collaboration between AI and humans becomes an opportunity to accumulate valuable data about correct actions and the intricate contexts surrounding them. This accumulation, in turn, becomes the cornerstone for the AI’s ongoing learning and refinement process.
Looking ahead, the undeniable truth remains that human understanding of the environment in which AI operates holds a distinct edge. This realization paves the way for the “experiential AI” model – a synergy combining human supervision and algorithms’ insights. While AI augments and streamlines processes, this collaborative approach ensures that human expertise and oversight remain integral for holistic and effective AI integration.