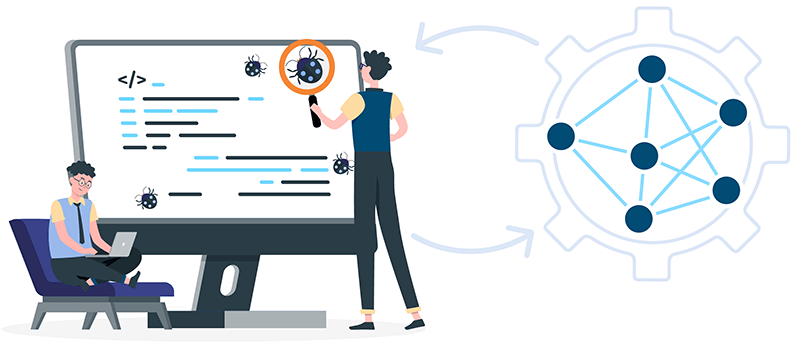
Machine learning (ML) models are an integral part of many modern applications, ranging from image recognition to natural language processing. However, developing and training ML models can be a complex and time-consuming process, and debugging and profiling these models is often a challenge. In this article, we will explore some tips and best practices for debugging and profiling ML model training.
Understand and prepare the data
Before diving into debugging and profiling, it is important to understand the data that is being used to train the ML model. This includes the format, size, and distribution of the data, as well as any potential biases or anomalies that may be present. Understanding the data can help to determine potential issues and inform decisions about preprocessing and feature engineering. Prepare the data to use only relevant information for model training.
Start with a simple model
When beginning the development process, it is often helpful to start with a simple model and gradually increase its complexity. This can help identify potential issues early on and make debugging and profiling easier. Once a simple model is working as expected, additional complexity can be added incrementally.
Check for data issues
Data issues can be a common cause of ML model errors. These issues can include missing data, inconsistent data formatting, and data outliers. It is important to thoroughly check the data for issues and preprocess it as necessary to ensure that the model is working with clean and consistent data.
Check for overfitting
Overfitting occurs when a model performs well on the training data but poorly on new, unseen data. Overfitting can be a common issue in ML model training, particularly when the model is complex or the training data is limited. To check for overfitting, it is important to split the data into training and validation sets and monitor the model’s performance on both sets.
Monitor training progress
Monitoring the training progress of the ML model can help to identify potential issues early on. This includes tracking metrics such as accuracy, loss, and convergence rate over time. If the model is not performing as expected, adjustments can be made to the model architecture, hyperparameters, or data preprocessing.
Use visualization tools
Visualization tools can be helpful for understanding the behavior of an ML model and identifying potential issues. These tools can include scatter plots, histograms, and heat maps. Visualization tools can also be used to visualize the model’s internal representations and activations, which can provide insight into how the model is processing the data. For instance, OptScale, a FinOps and MLOps open source platform, gives full transparency and a deep analysis of internal and external metrics to identify training issues. OptScale provides visualization of the entire ML/AI model training process, captures ML/AI metrics and KPI tracking, and helps identify complex issues in ML/AI training jobs.
Profile the model
Profiling the ML model can help detect potential bottlenecks and areas for optimization. This includes profiling the model’s computational performance, memory usage, and I/O operations. Profiling tools can help to identify areas where the model is spending the most time and suggest potential optimizations. Profiling tools like OptScale profile machine learning models and collect a holistic set of internal and external performance and model-specific metrics, which help identify bottlenecks, and give performance and cost optimization recommendations.
Use transfer learning
Transfer learning is a technique that involves leveraging the knowledge learned from one ML model to improve the performance of another. Transfer learning can be particularly useful when working with limited data or when developing complex models. By using a pre-trained model as a starting point, transfer learning can help to speed up the training process and improve the overall performance of the model.
Use automated hyperparameter tuning
Hyperparameters are the variables that control the behavior of the ML model, such as the learning rate and batch size. Tuning these hyperparameters can be a time-consuming process and require significant trial and error. Automated hyperparameter tuning can help speed up the tuning process and identify optimal hyperparameter settings. ML/AI model training is a complex process, which depends on a defined hyperparameter set, hardware, or cloud resource usage. OptScale enhances ML/AI profiling process by getting optimal performance and helps reach the best outcome of ML/AI experiments.
Test the model on new data
Once the ML model has been developed and trained, it is important to test it on new, unseen data. This can help identify potential issues with the model’s generalization and ensure that it is working as expected in real-world scenarios.
💡 You might be also interested in our article ‘What are the main challenges of the MLOps process?’
Discover the challenges of the MLOps process, such as data, models, infrastructure, and people/processes, and explore potential solutions to overcome them → https://hystax.com/what-are-the-main-challenges-of-the-mlops-process.
✔️ OptScale, a FinOps & MLOps open source platform, which helps companies optimize cloud costs and bring more cloud usage transparency, is fully available under Apache 2.0 on GitHub → https://github.com/hystax/optscale.