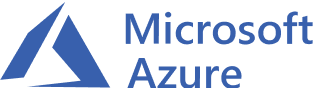
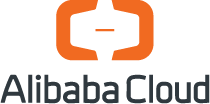
ML/AI model training tracking & profiling, internal/external performance metrics
Granular ML/AI optimization recommendations
Runsets to identify the most efficient ML/AI model training results
Spark integration
OptScale profiles machine learning models and analyzes internal and external metrics deeply to identify training issues and bottlenecks.
ML/AI model training is a complex process that depends on a defined hyperparameter set, hardware, or cloud resource usage. OptScale improves ML/AI profiling process by getting optimal performance and helps reach the best outcome of ML/AI experiments.
OptScale provides full transparency across the whole ML/AI model training and teams process and captures ML/AI metrics and KPI tracking, which help identify complex issues in ML/AI training jobs.
To improve the performance OptScale users get tangible recommendations such as utilizing Reserved/Spot instances and Saving Plans, rightsizing and instance family migration, detecting CPU/IO, IOPS inconsistencies that can be caused by data transformations, practical usage of cross-regional traffic, avoiding Spark executors’ idle state, running comparison based on the segment duration.
OptScale enables ML/AI engineers to run many training jobs based on a pre-defined budget, different hyperparameters, and hardware (leveraging Reserved/Spot instances) to reveal the best and most efficient outcome for your ML/AI model training.
OptScale supports Spark to make Spark ML/AI task profiling process more efficient and transparent. A set of OptScale recommendations, delivered to users after profiling ML/AI models, includes avoiding Spark executors’ idle state.
A full description of OptScale as a FinOps and MLOps open source platform to optimize cloud workload performance and infrastructure cost. Cloud cost optimization, VM rightsizing, PaaS instrumentation, S3 duplicate finder, RI/SP usage, anomaly detection, + AI developer tools for optimal cloud utilization.
Discover our best practices:
Find out how to:
1250 Borregas Ave, Sunnyvale, CA 94089, USA | [email protected]