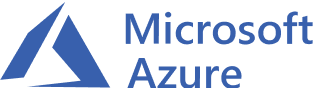
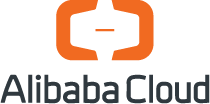
Rastreamento e perfil de treinamento de modelo ML/AI, métricas de desempenho internas/externas
Recomendações granulares de otimização de ML/IA
Runsets para identificar os resultados de treinamento do modelo ML/AI mais eficientes
Integração do Spark
O OptScale traça perfis de modelos de aprendizado de máquina e analisa profundamente métricas internas e externas para identificar problemas e gargalos de treinamento.
O treinamento do modelo ML/AI é um processo complexo que depende de um conjunto definido de hiperparâmetros, hardware ou uso de recursos de nuvem. OptScale melhora o processo de criação de perfil de ML/IA, obtendo desempenho ideal e ajuda a alcançar o melhor resultado dos experimentos de ML/IA.
O OptScale fornece total transparência em todo o processo de treinamento e equipes do modelo de ML/IA e captura métricas de ML/IA e rastreamento de KPI, que ajudam a identificar problemas complexos em trabalhos de treinamento de ML/IA.
Para melhorar o desempenho, os usuários do OptScale obtêm recomendações tangíveis, como utilização de instâncias reservadas/spot e planos de economia, dimensionamento de direitos e migração de família de instâncias, detecção de CPU/IO, inconsistências de IOPS que podem ser causadas por transformações de dados, uso prático de tráfego inter-regional, evitando Estado ocioso dos executores do Spark, executando comparação com base na duração do segmento.
O OptScale permite que os engenheiros de ML/IA executem muitos trabalhos de treinamento com base em um orçamento predefinido, diferentes hiperparâmetros e hardware (aproveitando instâncias reservadas/spot) para revelar o melhor e mais eficiente resultado para o treinamento do seu modelo de ML/AI.
OptScale oferece suporte ao Spark para tornar o processo de criação de perfil de tarefas do Spark ML/AI mais eficiente e transparente. Um conjunto de recomendações OptScale, entregues aos usuários após a criação de perfil de modelos de ML/AI, inclui evitar o estado ocioso dos executores do Spark.
Uma descrição completa do OptScale como uma plataforma de código aberto FinOps e MLOps para otimizar o desempenho da carga de trabalho na nuvem e o custo da infraestrutura. Otimização de custos de nuvem, redimensionamento de VM, instrumentação PaaS, localizador de duplicatas S3, uso de RI/SP, detecção de anomalias, + ferramentas de desenvolvedor de IA para utilização ideal da nuvem.
Conheça nossas melhores práticas:
Descubra como:
1250 Borregas Ave, Sunnyvale, CA 94089, EUA | [email protected]