In this article, we describe Process 2 – Feature engineering, or the development of features.
Por favor, encontre o esquema completo, que descreve os principais processos de MLOps aqui. As principais partes do esquema são blocos horizontais, dentro dos quais os aspectos procedimentais dos MLOps são descritos. Cada um deles é projetado para resolver tarefas específicas dentro da estrutura de garantir a operação ininterrupta dos serviços de ML da empresa.
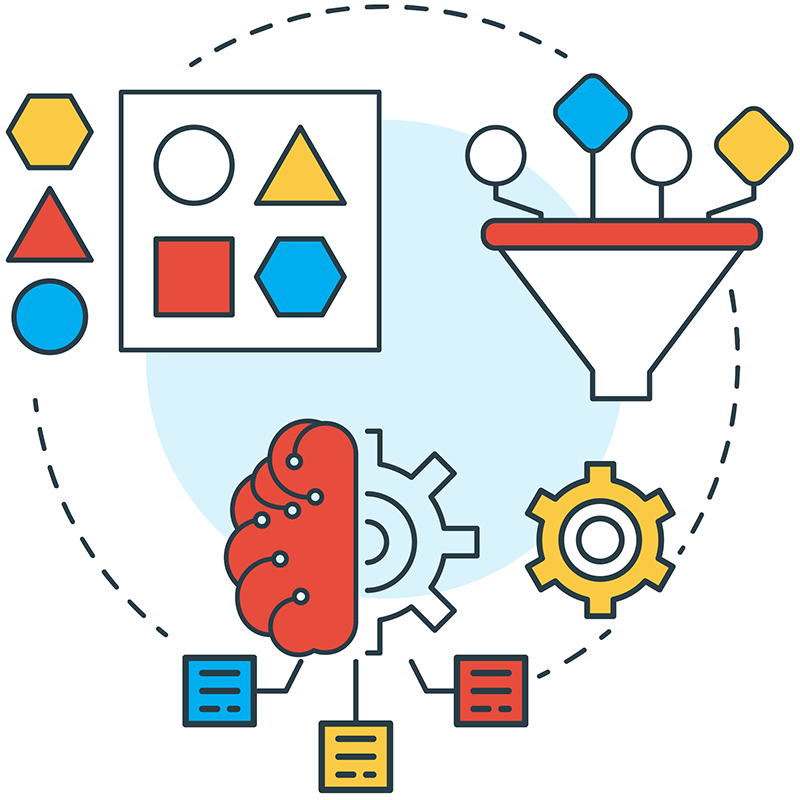
If desired metrics of an ML model cannot be achieved, one can try to expand the feature description of dataset objects with new features. By doing so, the context for the model will expand, and thus the desired metrics may improve.
New features can include:
- for tabular data: arbitrary transformations of existing object features, such as X^2, SQRT(X), Log(x), X1*X2, etc.,
- based on the subject area: body mass index, number of overdue credit payments per year, movie rating on IMDb, etc.
Let’s look at the part of the scheme that relates to feature engineering.
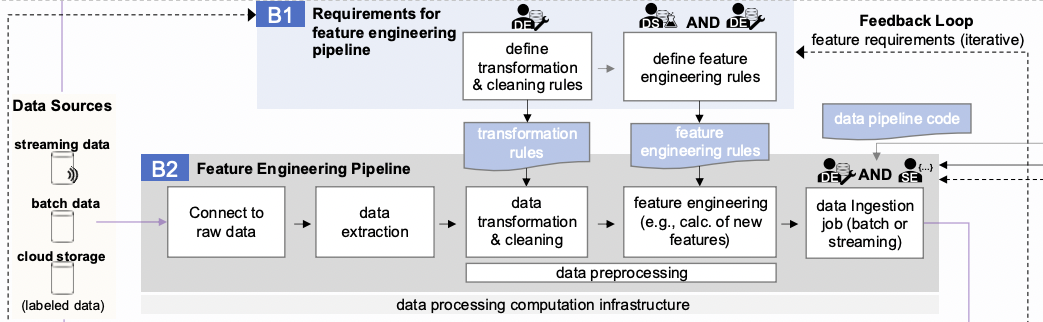
Block B1 is aimed at forming a set of requirements for transforming existing source data and obtaining features from them. The calculation of the features themselves is based on these pre-processed and cleaned data, according to the formulas introduced by the ML developer.
It is important to note that the feature engineering process is iterative. Applying one set of features may lead to new ideas, which are implemented in another set of features, and so on indefinitely. This is clearly shown in the scheme as a Feedback Loop.
The process of adding new features to the data is described in block B2 and includes:
- connecting to raw data,
- data extraction,
- data transformation and cleaning,
- feature engineering,
- and data ingestion.
Connecting to raw data
Connecting to raw data and data extraction are technical operations that can be quite challenging. For simplicity, let’s assume that there are several sources to which the team has access and tools for extracting data from these sources (at least Python scripts).
Data transformation and cleaning
This stage is almost identical to the similar step in the experiment block (C) – data preparation. In fact, at the stage of the first experiments, there is an understanding of what data and in what format is needed for ML model training. It remains only to generate and test new features correctly, but the data preparation process for this should be automated as much as possible.
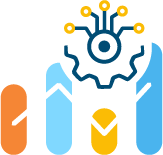
Otimização gratuita de custos de nuvem e gerenciamento aprimorado de recursos de ML/IA para toda a vida
Feature engineering
As noted above, these actions may consist of simply transforming several elements of the data tuple. Another option is to run a separate large processing pipeline to add one feature to the same tuple. In any case, there is a set of actions that are performed sequentially.
Data ingestion
The result of the previous actions is added to the dataset. Features are most often added to the dataset in batches to reduce the load on the databases. But sometimes it is necessary to do this on the fly (streaming) to speed up the execution of business tasks.
It is important to use the obtained features as efficiently as possible: to save and reuse them in the tasks of other ML developers in the company. For this purpose, there is a Feature store in the scheme. Ideally, it should be available within the company, separately administered, and versioned for all features entering it. The Feature store itself has two parts: online (for streaming scenarios) and offline (for batch scenarios).
Few will want to do all of this manually every time, especially since all the actions seem easily automated.
💡 You might be also interested in our article ‘Key MLOps processes (part 1): Experimentation, or the process of conducting experiments’ → https://hystax.com/key-mlops-processes-part-1-experimentation-or-the-process-of-conducting-experiments.
✔️ OptScale, uma plataforma de código aberto FinOps & MLOps, que ajuda empresas a otimizar custos de nuvem e trazer mais transparência ao uso da nuvem, está totalmente disponível no Apache 2.0 no GitHub → https://github.com/hystax/optscale.