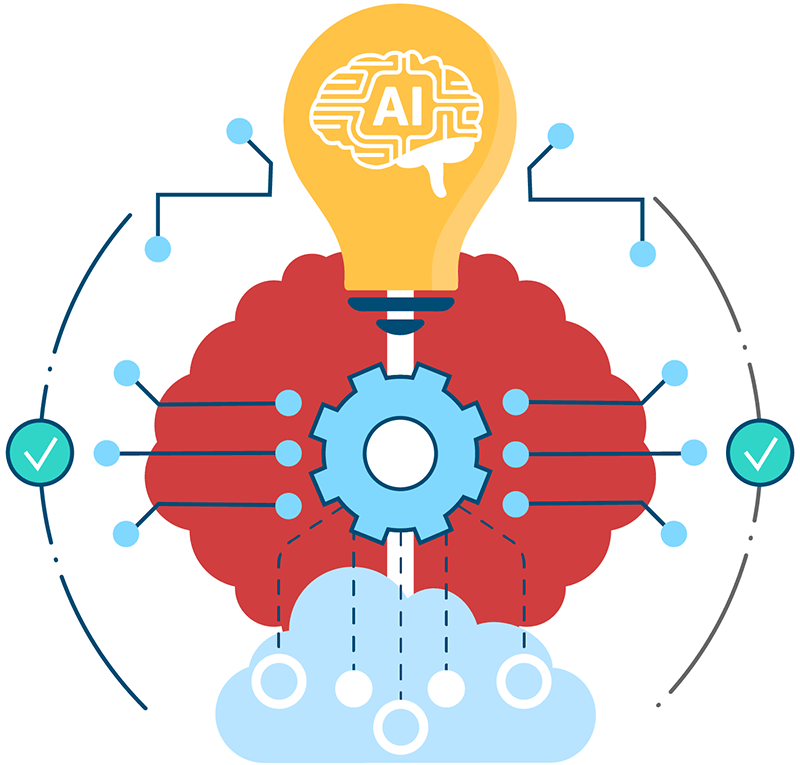
Managing cloud resources, particularly in allocation, has evolved into a complex and time-consuming endeavor. Challenges such as navigating the pitfalls of over or under-provisioning, addressing inefficiencies within cloud operations, establishing a cohesive authorization framework for multiple service providers, and contending with the heterogeneous nature of resources pose obstacles for IT professionals in achieving optimal cloud resource allocation. More than 30% of cloud expenditure is squandered due to suboptimal resource allocation practices. Complicating matters further, IT teams engage in manual resource provisioning to preempt delays and system crashes, a measure taken to ensure overall stability. Additionally, the periodic reassessment of cloud resources becomes imperative with each new version release to forestall unnecessary over-provisioning.
The extensive commitment to managing and fine-tuning resources diverts the attention of IT teams from their primary focus on delivering tangible business value. Such misalignment of priorities has the potential to impede companies’ strides in innovation. A promising avenue for addressing these challenges lies in leveraging the capabilities of Artificial Intelligence (AI) and Machine Learning (ML) to govern and optimize cloud resources. AI-driven cloud management offers a transformative solution, empowering IT teams to streamline the provisioning, monitoring, and optimization processes efficiently. This progressive approach warrants a closer examination to comprehend its potential impact.
The role of ML/AI
Mitigate cloud resource waste, enhance efficiency, and optimize expenditures
Enhance resource utilization through immediate automated responses
The manual monitoring of resources around the clock, even with a team of skilled IT specialists, is impractical. Artificial Intelligence/Machine Learning (AI/ML) provides a continuous monitoring capability, enabling real-time responses to sudden surges in computing resource demand. This entails the automatic allocation of resources to meet increased demand and scaling down when resources are not needed. Furthermore, AI/ML offers a detailed overview of resource allocation, empowering IT teams to enhance efficiency. This capability aids in the identification of inefficiencies and bottlenecks, enabling adjustments to improve scalability and prevent unnecessary downtimes.
Streamline resource optimization through Automation
Navigating the ebb and flow of fluctuating demands poses a perennial challenge for IT teams seeking to optimize cloud resources promptly. The specter of potential system crashes, unplanned downtimes, and suboptimal customer experiences looms large. A transformative solution lies in the hands of Machine Learning/Artificial Intelligence (ML/AI), where automation takes center stage to redefine resource optimization.
- AI takes the lead in managing resources and overseeing provisioning and adjustments.
- It operates through continuous 24/7 monitoring, detailed analysis, and instant reporting.
- The system’s responsiveness allows for dynamic creation or retirement of instances.
- Resource allocation is finely tuned with meticulous precision.
- The result is an unmatched level of scalability for efficient operations.
- Embracing intelligent automation promises a new, seamless, influential resource management era.
Ensure proactive app maintenance for uninterrupted performance
Imagine the holiday season: a surge of customers engaging with your app, eagerly making diverse product purchases. Suddenly, the app crashes, leaving customers unable to place orders. Some patiently await resolution, while others abandon the app, losing customers and revenue. Within this unpredictable scenario, various factors may contribute to glitches. One such factor is the challenge of meeting the sudden surge in resource demand. Another issue is the difficulty of predicting potential cloud performance issues before they manifest. Fortunately, ML/AI presents innovative solutions to both challenges. The IT team utilizes ML/AI for in-depth historical and real-time data analyses.
Traffic prediction:
ML/AI is employed to predict traffic spikes accurately and determines the required storage and computing resources at various times to optimize app performance.
Anomaly detection:
ML/AI is pivotal in identifying anomalies or deviations and detecting irregularities that could impede optimal cloud function.
Proactive threat detection:
The power of ML/AI enables IT teams to detect threats and vulnerabilities proactively and mitigates risks associated with potential security breaches.
Enhanced application security:
By harnessing the capabilities of ML/AI, the application’s overall security is significantly enhanced.
ML/AI emerges as a transformative solution for these challenges, offering:
Resource efficiency unveiled: Leverage ML/AI to unveil instances of over-provisioned and under-utilized resources. Receive personalized recommendations to deactivate dormant assets, enhancing resource efficiency.
Dynamic cloud workload mastery: Embrace the power of automation as ML/AI dynamically optimizes and manages cloud workloads. Seamlessly adjusts to fluctuating demands, ensuring optimal performance and resource utilization.
Strategic budget empowerment: Harness the forecasting capabilities of ML/AI to predict cloud costs and potential overruns. Equip your IT teams with valuable insights for strategic resource allocation, empowering them to manage budgets effectively.
Proactive security safeguard: Fortify your cloud infrastructure against potential financial losses from security breaches. AI/ML is a vigilant guardian, providing continuous monitoring and proactive measures to safeguard valuable assets.
Embrace a future-forward approach with ML/AI-enhanced cloud resource optimization
In the dynamic realm of cloud management, where organizations increasingly migrate workloads, the intricacies of resource allocation have heightened. Traditional methods are insufficient for a landscape demanding innovation from IT teams, which risks diverting valuable time away from high-impact tasks. In contrast, a pivot from manual processes to ML/AI-driven solutions is imperative for optimizing cloud resource allocation.
Thus, as a worthy example, the Hystax OptScale platform and its latest MLOps enhancement is the Power Schedules feature. This addition offers advanced control over the efficient utilization of IT resources across various cloud service providers. As part of our continuous commitment to refining cloud efficiency and management, we have pinpointed a consistent demand from our customers for a more organized method to govern their IT resources.
✔️ Do you want your cloud and ML/AI operations to be under control and your expenses to meet your expectations? Assess the capabilities and potential of an open source platform OptScale → https://hystax.com/introducing-optscale-public-release-an-open-source-powerhouse-for-finops-and-mlops/
Hystax OptScale offers an MLOps and FinOps platform for cloud and ML/AI enthusiasts that is fully available under Apache 2.0 on GitHub → https://github.com/hystax/optscale