What is FinOps?
FinOps is a relatively new concept popular among IT leaders, aimed to optimize the cost of infrastructure, build efficient cloud usage and enhance close collaboration among engineering departments and managers. This daily joint work of several teams and departments is one of the main FinOps principles which reveals great optimization opportunities for a proper R&D process.
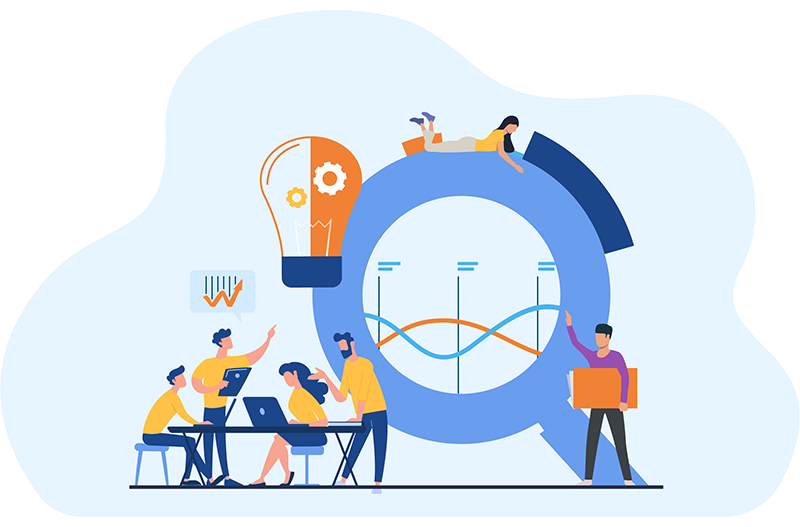
FinOps meaning, core principles and advantages that are ensured in case of its implementation are described in detail in a series of our previous articles:
FinOps goals
Spending thousands of dollars on IT infrastructure, businesses are eager to gain full transparency of spends, achieve satisfactory unit economics metrics and build a strong FinOps team of highly involved and motivated employees.
FinOps strategy helps eliminate a great amount of challenges on the way to achieve these goals. The main idea of FinOps is not only to cut cloud costs at once, but to build a flow that allows optimizing R&D processes, and increasing efficiency of public cloud usage.
The main FinOps goals might be defined as the following:
- Optimize IT infrastructure expenses
- Identify waste of money and R&D processes bottlenecks
- Bring observability
- Establish a long-term cost-saving process
- Engage engineers in cost savings
FinOps team and responsibilities
Traditionally someone from a top management team worries about cloud expenses, cloud budget forecast and its optimization – it might be a CFO if there is such a position or CTO, CIO & CEO in small and medium businesses. Typically these people own cloud budgets in a company: struggling with total mess in cloud bills and trying to create an accurate forecast of cloud spends.
There might be a dedicated FinOps leader, but in order to achieve maximum efficiency, it’s recommended to involve the following titles into FinOps and cloud cost optimization processes.
- Head of Finance (CFO) is responsible for the whole strategy, forecasting and FinOps reporting
- IT Manager might be responsible for full infrastructure transparency and observability, resource usage optimization
- Team leads are to create a smooth FinOps process among their teams
- Architects, DevOps and Engineers are responsible for their proper resource usage
Engineers generate a major part of every cloud bill, but their participation in the budget optimization process is underestimated. One of the most popular challenges is to involve engineers in a cost-saving process. They are motivated to close tickets in Jira and don’t care about budget allocation.
How to engage engineers in FinOps and cloud cost savings
It’s efficient to ask engineers to do regular resource clean ups, deactivate unnecessary resources (volumes, AMIs, snapshots, etc.), check VM rightsizing and focus on reserved instances and some saving recommendations. This simple routine can help optimize cloud bills at once, but it can’t establish a system of proper resource usage and FinOps implementation.
When your engineering department is an integral part of a FinOps team, you’ll get improved R&D efficiency with a minimal and predictable infrastructure cost.
On daily basis they can help:
- Organize applications and resource usage – implement a convenient tagging system to assist with budget allocation. Map all cost among projects, teams and business goals
- Optimize expenses for cloud and K8s workloads
- Follow budget constraints and get real-time alerts about budget exceeds
OptScale is a solution to enhance FinOps teamwork and speed up FinOps implementation. It helps organize shared workload usage, optimize & forecast Kubernetes and cloud costs, engage engineers in cost savings. OptScale offers:
- AWS, Microsoft Azure, Alibaba cloud cost optimization
- K8s cost optimization
- Resource optimization for CI/CD jobs
- R&D cost allocation and cost delivery forecast
- IT environment management: shared environment planning and booking
- Cloud health monitoring
Are you struggling with cloud spend control? Test Environment Management helps tackle numerous challenges almost every modern tech company faces → ‘In our recent article find out how proper Test Environment Management helps achieve cloud cost optimization goals.’